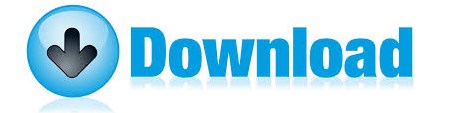
How do data become clustered? One way is to collect data on the same subjects over time. Please see Harrell (2015) and Scariano and Davenport (1987) for explanations and examples. If this assumption is violated by having clustering in the data, the standard errors around the point estimates will be underestimated, and false alarms will be more likely. The effects of violating this assumption depend on how the assumption is violated. From a practical stand point, this means that each observation is represented on one and only one row in the data file. Remember that one of the most important assumptions of OLS regression is that the observations are independent.
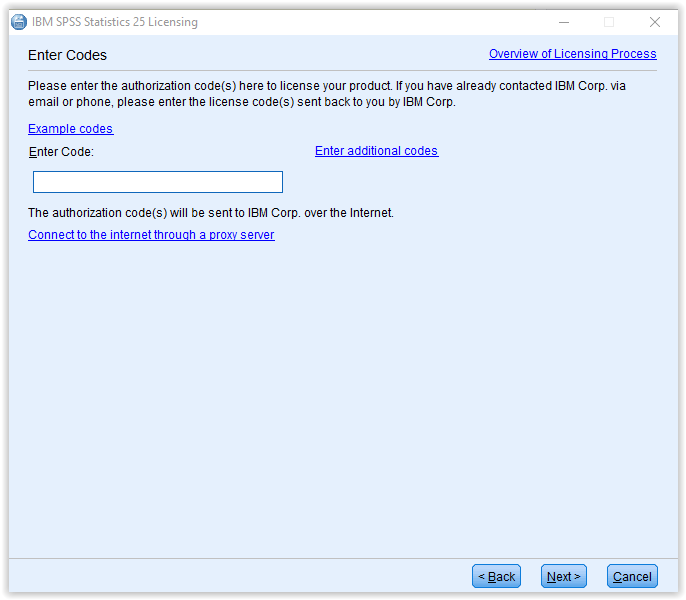
Obviously, there are already several ways to run an OLS regression in SPSS, so what else can the mixed command do? It can run linear models with clustered data. The print subcommand is used to have the parameter estimates included in the output (although the options used on the subcommand are different). The SPSS keyword with is used with both the glm and the mixed commands to indicate that the two predictor variables, read and female, are to be treated as continuous.
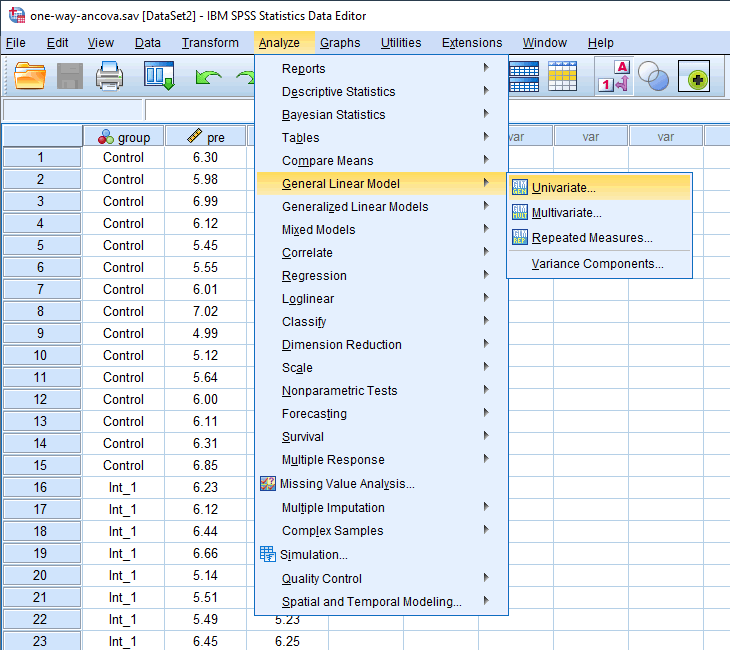
Here is the same model run with the mixed command. How would you run a linear regression model in SPSS? Perhaps you would use either the regression command or the glm command. In this type of regression, the outcome variable is continuous, and the predictor variables can be continuous, categorical, or both. When most people think of linear regression, they think of ordinary least squares (OLS) regression. The mixed command in SPSS is used to run linear regression models, including mixed effects models. References will be provided so that those interested in these topics can find additional information. Because the purpose of this workshop is to show the use of the mixed command, rather than to teach about multilevel models in general, many topics important to multilevel modeling will be mentioned but not discussed in detail. Such models are often called multilevel models.
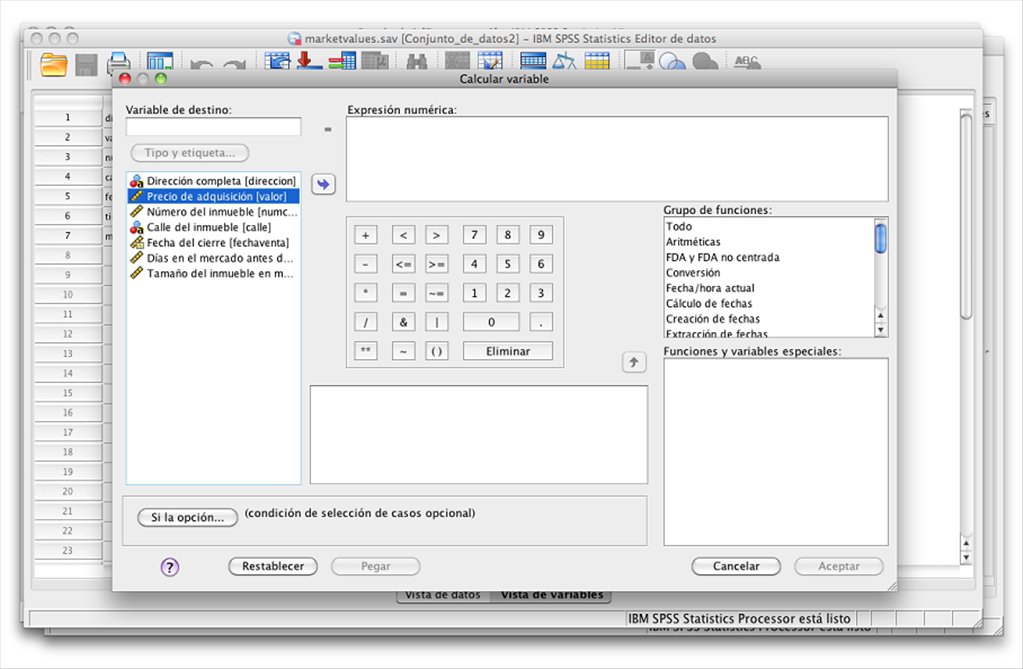
Although it has many uses, the mixed command is most commonly used for running linear mixed effects models (i.e., models that have both fixed and random effects). The purpose of this workshop is to show the use of the mixed command in SPSS. Using the SPSS Mixed Command Introduction
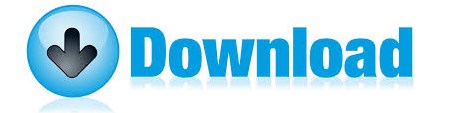